Navigating Accents and Dialects in AI Voice Bots: Challenges and Innovations
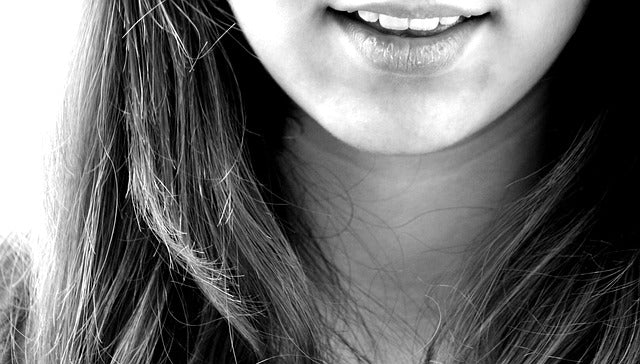
Introduction
In an increasingly globalized world, the ability of AI voice bots to understand and replicate diverse accents and dialects is crucial for effective communication. Languages like English and German exhibit significant regional variations, posing challenges for AI systems in accurately recognizing and generating speech. This article explores the complexities associated with accents and dialects in AI voice bots, examines current capabilities, and discusses potential solutions to enhance their performance.
Understanding Accents and Dialects
Accents refer to variations in pronunciation within a language, often influenced by regional or social factors. Dialects encompass broader linguistic differences, including vocabulary and grammar, specific to a particular group. For instance, English encompasses accents such as American, British, Irish, and Singaporean, each with distinct phonetic characteristics. Similarly, German is spoken with varying accents and dialects in Germany, Switzerland, and Austria.
Challenges in AI Speech Recognition
AI voice bots rely on Automatic Speech Recognition (ASR) systems to transcribe spoken language into text. These systems are typically trained on standard language datasets, which may not encompass the full spectrum of regional accents and dialects. Consequently, ASR systems often struggle with:
-
Phonetic Variations: Different accents may alter vowel and consonant sounds, leading to misinterpretation.
-
Prosodic Differences: Variations in intonation, stress, and rhythm can affect comprehension.
-
Lexical Disparities: Dialects may include unique vocabulary not present in standard language models.
These challenges can result in decreased accuracy and user satisfaction, particularly for speakers with less common accents.
Advancements in Accent and Dialect Recognition
To address these challenges, researchers and developers are employing several strategies:
-
Diverse Training Data: Incorporating speech samples from various accents and dialects enhances ASR robustness. For example, voice bots can understand different English accents like British, American, Australian, Indian, and French by utilizing diverse speech data and advanced Natural Language Understanding (NLU) techniques.
-
Dialect Identification Systems: Implementing systems that first identify a speaker's dialect can enable the selection of appropriate models for accurate transcription. For instance, a dialect identification system can infer the dialect of the speaker to use adapted dialectal speech recognition models, improving transcription quality.
-
Acoustic Model Adaptation: Adjusting models to account for specific phonetic features of different accents improves recognition accuracy. This approach involves training acoustic models with data that reflects the phonetic variations of target accents.
-
Pronunciation Lexicons: Developing comprehensive lexicons that include regional pronunciations aids in accurate speech recognition. These lexicons map words to their phonetic representations across different dialects.
Case Study: Swiss German Dialects
Swiss German presents a unique challenge due to its significant deviation from Standard German. Efforts to build systems that translate High German text to Swiss German speech in different dialects involve creating datasets and deep learning models for machine translation and speech synthesis. Such projects aim to develop voice assistants capable of understanding and speaking Swiss German, thereby enhancing accessibility for Swiss German speakers.
Text-to-Speech (TTS) and Accent Generation
Beyond recognition, generating speech in various accents is vital for applications like language learning and personalized user interactions. Advanced TTS systems now offer a range of AI voices with different accents, allowing users to select preferred speech patterns. For instance, platforms provide comprehensive lists of AI voices and languages featuring various accents, enabling the creation of more relatable and engaging content.
Implications for Multilingual Voice Bots
Multilingual voice bots are designed to interact with users across different languages and accents. Leveraging Natural Language Processing (NLP) and machine learning, these bots can comprehend and respond to queries in multiple languages, seamlessly transitioning between them within the same conversation. This capability enhances user experience by providing accessible and inclusive interactions.
Challenges and Considerations
Despite advancements, several challenges persist:
-
Resource Limitations: Accents and dialects with limited available data pose difficulties for model training.
-
Computational Complexity: Supporting multiple accents increases the complexity and resource requirements of AI systems.
-
Continuous Evolution: Languages and accents evolve over time, necessitating ongoing updates to language models.
Future Directions
Ongoing research aims to develop more inclusive and robust AI voice bots capable of handling a wide array of accents and dialects. Innovations such as accent-specific codebooks and world-English language models for on-device virtual assistants are being explored to enhance performance across diverse speech patterns.
Conclusion
The ability of AI voice bots to accurately recognize and generate speech across various accents and dialects is essential for effective communication in a diverse world. While challenges remain, ongoing advancements in data collection, model adaptation, and linguistic research continue to enhance the inclusivity and performance of AI-driven speech technologies.