Wie KI-Telefonbots mit mehreren Sprachen, Dialekten und branchenspezifischen Begriffen umgehen
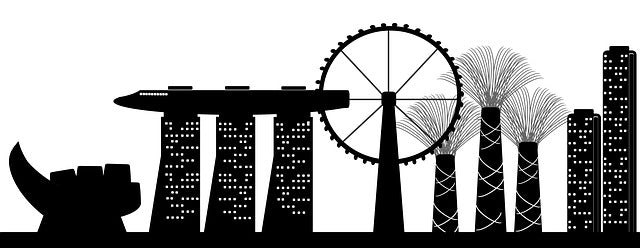
KI-Telefonbots sind unverzichtbare Werkzeuge für die Callcenter-Branche geworden, da sie Effizienz und Skalierbarkeit im Kundenservice bieten. Eine der größten Herausforderungen, mit denen sie konfrontiert sind, besteht jedoch darin, die Komplexität verschiedener Sprachen, Dialekte und Fachbegriffe, die in verschiedenen Branchen oder spezifischen Organisationen verwendet werden, zu bewältigen. Für Callcenter-Profis ist es entscheidend, zu verstehen, wie diese Bots funktionieren und welche Ressourcen für ihre erfolgreiche Implementierung erforderlich sind.
In diesem Artikel untersuchen wir, wie KI-Telefonbots sprachliche Nuancen wie britisches und amerikanisches Englisch, Singlish und Branchenslang handhaben, während wir auch die benötigte Manpower, Fähigkeiten und den Zeitrahmen für die Einführung dieser Systeme ansprechen.
1. Die Herausforderung mehrerer Sprachen und Dialekte
1.1 Verständnis von Sprachen und Dialekten
-
Sprachvarianten: Unterschiede zwischen britischem Englisch (z.B. "lift") und amerikanischem Englisch (z.B. "elevator") können Benutzer verwirren, wenn sie nicht angesprochen werden.
-
Singlish: Einzigartig für Singapur kombiniert Singlish englische, chinesische, malaiische und tamilische Ausdrücke (z.B. "Can lah" oder "No need already").
1.2 Der Ansatz der KI zur Handhabung von Sprache und Dialekten
-
NLP-Modelle: Modelle der natürlichen Sprachverarbeitung (NLP) werden auf regionalen Datensätzen trainiert, um lokale Sprachnuancen zu erkennen.
-
Akzent- und Ausspracheerkennung: KI-Systeme nutzen maschinelles Lernen, um Akzente und Aussprachen zu unterscheiden.
-
Echtzeit-Anpassung: Fortschrittliche Algorithmen passen sich während Gesprächen an die Akzente und Sprachpräferenzen der Sprecher an.
1.3 Häufige Herausforderungen
-
Gemischte Sprachsätze: Erkennung von Phrasen, die Sprachen mischen, wie das Singlish, das Englisch und Chinesisch kombiniert.
-
Regionale Unterschiede: Variationen in Aussprache und Verwendung, wie "petrol" im Vereinigten Königreich im Vergleich zu "gas" in den USA.
2. Verwaltung von branchenspezifischen und organisatorischen Begriffen
2.1 Bedeutung spezialisierter Begriffe
-
Branchenslang: Begriffe wie "Fall-ID" im technischen Support oder "Selbstbehalt" in der Versicherung benötigen präzise Erkennung.
-
Organisatorische Terminologie: Unternehmen verwenden häufig proprietäre Akronyme und workflowbezogene Begriffe.
2.2 KI-Training für spezialisiertes Vokabular
-
Benutzerdefinierte Datensätze: KI wird auf Datensätzen trainiert, die branchenspezifische und organisationsspezifische Begriffe enthalten.
-
Simulierte Umgebungen: Bots werden in simulierten Kundenszenarien getestet, um die Begriffsrecognition zu verfeinern.
-
Kontinuierliches Lernen: KI-Systeme aktualisieren ihre Wissensbasis, wenn neue Begriffe eingeführt werden.
2.3 Mögliche Fallstricke
-
Mehrdeutigkeit: Ähnliche Begriffe in verschiedenen Branchen (z.B. "Konto" im Bankwesen vs. E-Commerce).
-
Datenvolumen: Große Wortschätze können die Ressourcen der KI belasten, was ein effizientes Datenmanagement erfordert.
3. Bereitstellung: Ressourcen, Teams und Zeitpläne
3.1 Fähigkeiten erforderlich
-
Datenwissenschaftler: Trainieren und verfeinern von NLP- und Machine-Learning-Modellen.
-
Branchenexperten: Bereitstellung von Fachwissen für spezialisierte Terminologie.
-
IT-Ingenieure: Verwaltung von Systemintegration, Infrastruktur und Leistung.
-
UX/UI-Designer: Sicherstellen, dass der Bot eine benutzerfreundliche Erfahrung bietet.
3.2 Teamgröße
-
Kleinmaßstäbliche Bereitstellung: Erfordert 5–10 Teammitglieder, die sich auf ein oder zwei Sprachen konzentrieren.
-
Großangelegte Bereitstellung: Umfasst 20–50 Mitglieder, unterstützt mehrere Sprachen und spezialisierte Branchen.
3.3 Zeitpläne
-
Kleine Projekte: Dauern typischerweise 3–6 Monate.
-
Komplexe Implementierungen: Können 12–18 Monate in Anspruch nehmen, insbesondere bei mehrsprachigen und branchenspezifischen Integrationen.
4. Erfolgsgeschichten
4.1 Umgang mit Dialekten im Englischen
-
Reisebranche: KI-Bots verwalten erfolgreich sowohl britisches als auch amerikanisches Englisch, um globalen Reisenden bei Buchungen und Änderungen von Reiserouten zu helfen.
4.2 Branchenspezifische Anwendungen
-
Gesundheitswesen: Bots in medizinischen Callcentern erkennen Begriffe wie "Eigenanteil" und "Selbstbehalt", was Verwirrung bei den Patienten reduziert.
4.3 Anpassung an Singlish
-
Ein Singlish-fähiger Bot, der in Singapur eingesetzt wurde, verbesserte die Kundenzufriedenheit, indem er lokale Phrasen und den Ton verstand.
5.Zukünftige Perspektiven
5.1 Multimodale KI-Integration
-
Kombination von Sprach-, Text- und visuellen Eingaben für umfassendere Interaktionen.
-
Beispiel: Bots, die Textbestätigungen für gesprochene Anweisungen bereitstellen.
5.2 Echtzeit-Lernen
-
Kontinuierliche Anpassung an neue Sprachen, Phrasen und Fachbegriffe während Live-Interaktionen.
5.3 Globale Standardisierung
-
Anpassung an internationale Vorschriften und Sprachen zur Schaffung universell anwendbarer Systeme.
6. Schlussfolgerung
KI-Telefonbots revolutionieren die Callcenter-Branche, indem sie verschiedene Sprachen, Dialekte und Fachbegriffe verarbeiten. Obwohl diese Bots leistungsstark sind, erfordert ihre erfolgreiche Implementierung ein qualifiziertes Team, Zeit und sorgfältige Planung.
Durch das Verständnis der Fähigkeiten und Einschränkungen dieser Systeme können Callcenter-Profis sicherstellen, dass KI-Bots den sich entwickelnden Bedürfnissen mehrsprachiger und branchenübergreifender Kundenstämme gerecht werden. Mit dem Fortschritt der Technologie werden Bots noch leistungsfähiger, was es Organisationen ermöglicht, nahtlose, global inklusive Kundenerlebnisse zu bieten.